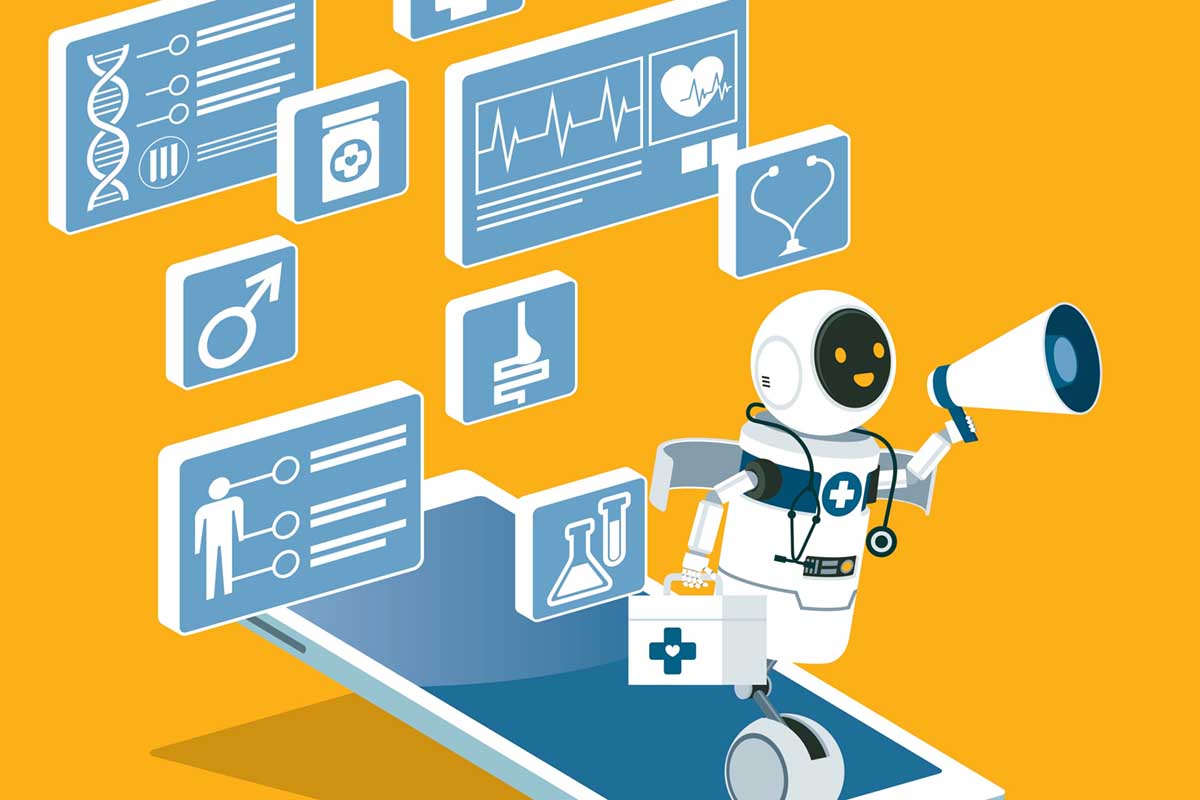
Artificial Intelligence (AI) promises a revolution in healthcare, and the revenue cycle is a prime target for its transformative potential. However, implementing AI effectively in RCM presents unique challenges that can’t be ignored. Here’s a closer look at the roadblocks on the path to a fully AI-powered revenue cycle:
Data Hurdles:
- Data Quality: AI thrives on clean, complete data; however the electronic health record (EHR) is far from clean (e.g. one analysis found that half the content of an EHR was copied forward). Inconsistent documentation, coding errors, and missing information in medical records can significantly hinder the accuracy and effectiveness of AI algorithms.
- Data Interoperability: Healthcare data often resides in siloed systems, making it difficult for AI to access and integrate the information it needs to function optimally.
Algorithmic Obstacles:
- Complexity of Healthcare data: Medical coding and billing involve a vast array of rules, regulations, and nuances. Developing AI models that can navigate this complexity and handle edge cases effectively remains a challenge.
- Explainability Issues: AI algorithms can sometimes be “black boxes,” making it difficult to understand how they arrive at specific decisions. This lack of transparency can be problematic for regulatory compliance and provider trust.
Human Hurdles:
- Change Management: Transitioning to AI-powered RCM requires cultural change within healthcare organisations. Staff may need training and support to adapt to new workflows and overcome potential fears of job displacement.
- Integration Challenges: Integrating AI seamlessly with existing IT infrastructure can be complex and require significant upfront investment.
The Road Ahead: Overcoming the Obstacles
Despite these challenges, AI holds immense potential for RCM. Here’s what can bridge the gap:
- Focus on Data Quality: Improved data capture practices, standardisation, and data cleansing efforts are crucial for laying a solid foundation for AI.
- Open and Interoperable Systems: Standardising data formats and promoting interoperability between healthcare systems will allow AI to access the data it needs to function effectively.The adoption of data standards like FHIR and regulations like the 21st Century Cures Act are helping to break down data silos.
- Explainable AI Models: Developing AI models that provide clear explanations for their decisions will build trust and ensure regulatory compliance. LLMs actually offer an opportunity to make traditional AI systems more interpretable and user-friendly.
- Collaboration is Key: Successful AI implementation requires collaboration between healthcare providers, IT teams, and AI vendors, using the principles of human-centered designs.
Phare Health: Your Partner in AI-Powered RCM
While challenges exist, Phare Health is committed to helping you navigate the journey towards an AI-powered revenue cycle. We understand the importance of data quality and offer solutions that can help you clean and standardise your data. Additionally, our focus on explainable AI ensures transparency and builds trust in our solutions.
By partnering with Phare Health, you gain access to cutting-edge AI technology combined with a deep understanding of healthcare RCM. Together, we can overcome the hurdles and unlock the true potential of AI to optimise your revenue cycle and ensure long-term financial stability.